Zifei Nieさんの論文がApplied Energy誌に掲載されました!
Energy-efficient lane-change motion planning for personalized autonomous driving
The research paper of Zifei Nie (Q-ENERGY fellow, year 2022) has been published in the journal of Applied Energy, one of the top 10% journals in the energy field (Impact Factor = 11.46)!
Context and importance for decarbonization
The research proposes a novel lane-changing motion planning strategy for personalized energy-efficient autonomous driving. The strategy uses key technologies such as trajectory planning and tracking to implement energy-efficient, personalized, and safe mobility.
A driving style identification module is developed based on real driving data, and an energy-optimal lane-changing trajectory representing a specific driving style is found and used as a reference for real-time nonlinear model predictive control. Experimental verification demonstrated that the proposed strategy could achieve energy savings compared to human drivers’ maneuvers and satisfy human drivers’ personalized driving preferences.
This research is important for building a future decarbonization society as it provides a solution for energy-efficient and safe autonomous driving, contributing to reducing carbon emissions from transportation.
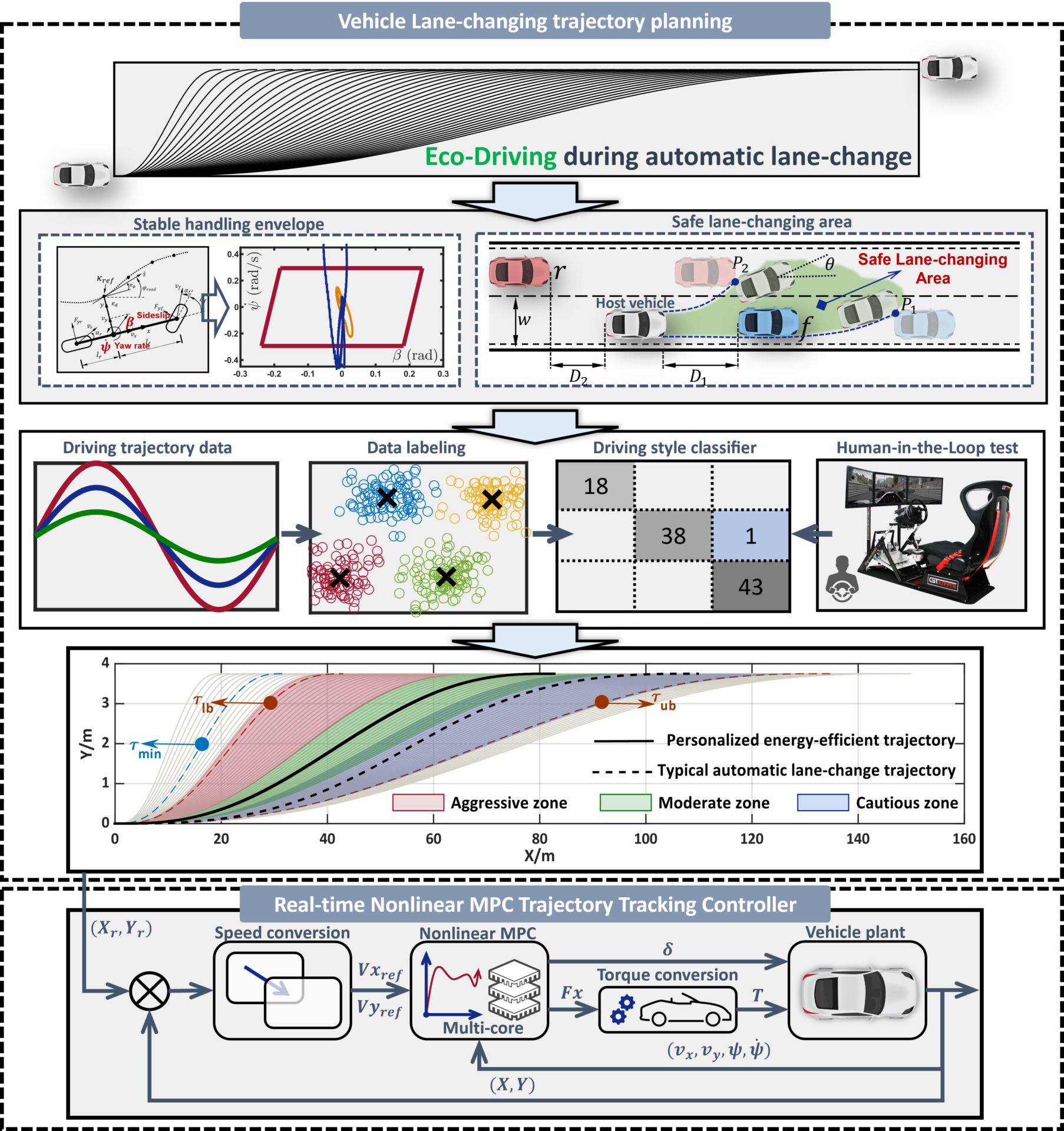
Abstract
With the aim of realizing energy-efficient, personalized, and safe mobility, a novel lane-changing motion planning strategy for personalized energy-efficient autonomous driving is proposed in this research. The key technologies consist of trajectory planning and trajectory tracking. Taking the quintic polynomials as the general trajectory cluster generator, the overall trajectory planning is converted into a constrained optimization problem using the lane-changing duration. The feasible and safe lane-changing trajectories can be extracted from the general trajectory cluster by introducing a stable handling envelope and a safe lane-changing area considering the constraints of vehicle dynamics limitation and surrounding traffic vehicles. A driving style identification module is developed based on multi-class Gaussian process classification utilizing real driving data to determine the trajectories that can characterize personalized features. Reflecting the constraints of feasibility, safety, and personalization on the boundaries of lane-changing duration, an energy-optimal lane-changing trajectory representing a specific driving style can be found and regarded as a reference. To precisely and rapidly control the vehicle to track the reference trajectory, a real-time nonlinear model predictive controller is designed and solved utilizing the parallel method. The algorithms proposed above are integrated and Driver-in-the-Loop experimental verifications are conducted. Experiment results demonstrated that the proposed strategy is able to realize lane change with an energy saving rate of 2.87% to 5.73% compared with human drivers’ maneuver. Comparative simulation with a typical automatic lane-change model also shows the effectiveness of the proposed approach, which is capable of not only accomplishing the energy-efficient lane change but also satisfying human driver’s personalized driving preferences.
Figure: System Architecture of the proposed personalized energy-efficient lane-changing motion planning.
The full version of the paper can be accessed using the following information.
Journal: Applied Energy
Title: Energy-efficient lane-change motion planning for personalized autonomous driving
Authors: Zifei Nie, Hooman Farzaneh
DOI:10.1016/j.apenergy.2023.120926